-
Notifications
You must be signed in to change notification settings - Fork 29
Home
Problem: A deeper understanding of the molecular drivers of disease, within the context of clinical phenotypes, is currently limited by our ability to integration and analyze clinical and molecular knowledge at scale.1,2,3
Solution: Knowledge graphs provide meaningful ways to integrate heterogeneous biological data and represent complex biological mechanisms.4
Objective: This work seeks to explore the utility of incorporating existing knowledge of molecular mechanism from ontologies, publicly available data, and the literature in order to build a biomedical knowledge graph that models unbiased molecular mechanisms of human disease.
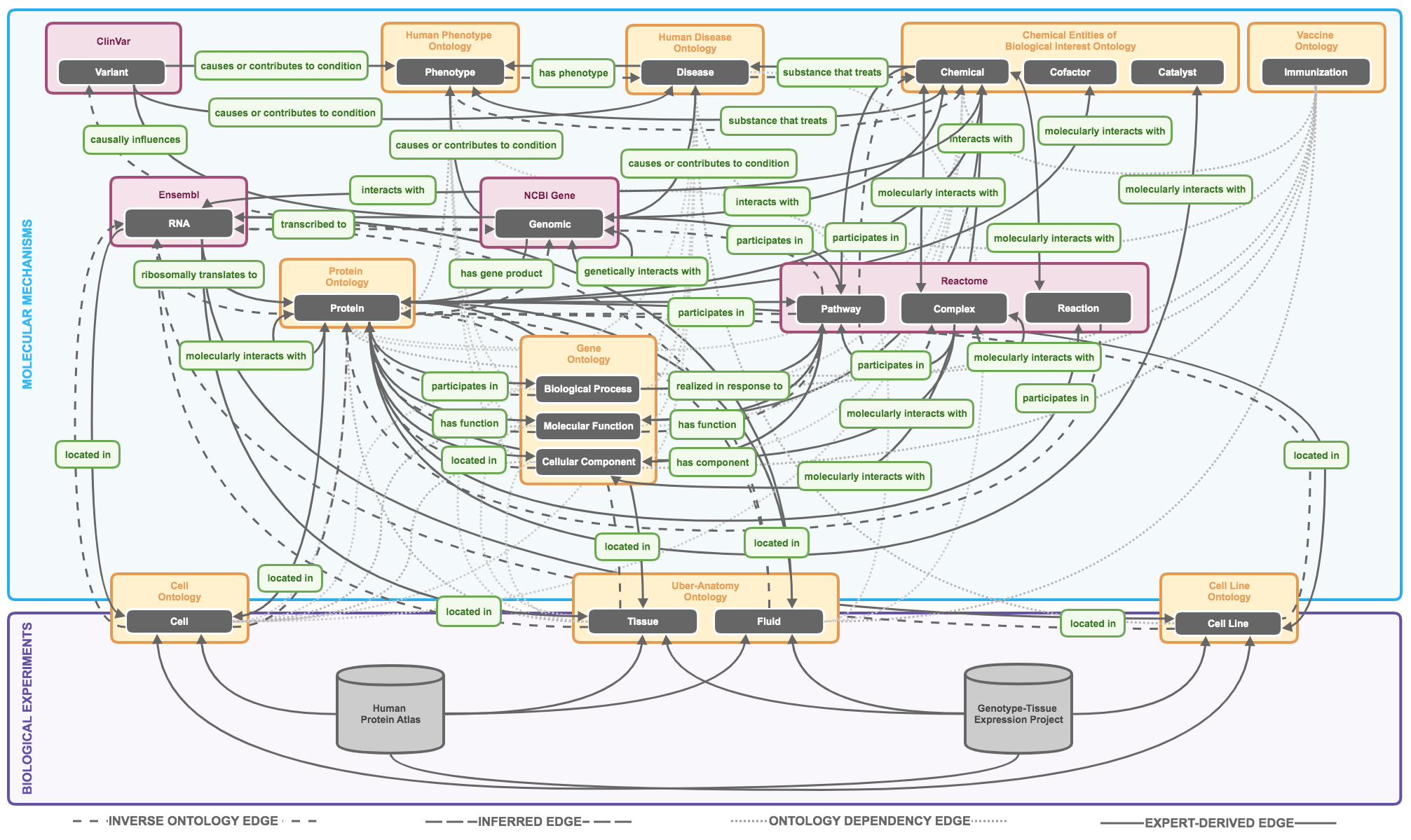
Translational Research Informatics Team
![]() |
![]() |
![]() |
![]() |
---|---|---|---|
Bill Baumgartner | Ignacio Tripodi | Adrianne L. Stefanski | Jordan Wyrwa |
The resulting knowledge graphs and molecular mechanism embeddings are free to download and included as part of each release.
- We presented this work (poster) at the 15th Annual Rocky Mountain Bioinformatics Conference held December 6-8th in Snowmass, Colorado.
- Ignacio Tripodi will present results on MechSpy, a novel application that uses PheKnowLator to perform toxicological mechanistic inference at the 2019 meeting of The American Society for Cellular and Computational Toxicology held September 24th-26th in Gaithersburg, Maryland.
- PheKnowLator is referenced in a review article on Knowledge-based Data Science in the biomedical domain.
We'd love to hear from you! To get in touch with us, please create an issue or send us an email 💌
This project is licensed under Apache License 2.0 - see the LICENSE.md file for details. If you intend to use any of the information on this Wiki, please provide the appropriate attribution by citing this repository:
@misc{callahan_tj_2019_3401437,
author = {Callahan, TJ},
title = {PheKnowLator},
month = mar,
year = 2019,
doi = {10.5281/zenodo.3401437},
url = {https://doi.org/10.5281/zenodo.3401437}
}